Business Analytics with Data Quality
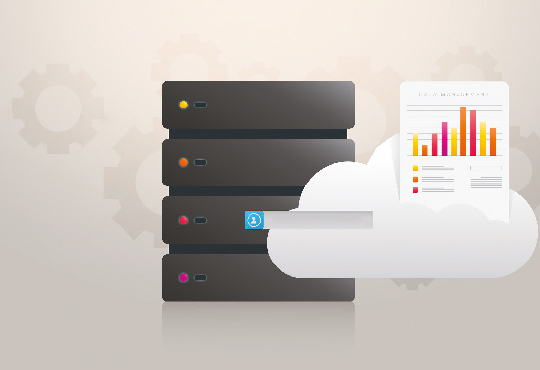
Abhishek Pal, Sr. Program Manager, Analytics & Business Partnership Global IT & Tata Communications
Today's economy is driven by data. Data and analytics capability ranks the highest of the top five investment priorities for CEOs today. It determines market trends, analyzes performance, and even presents insights that help to direct the future of the company. According to the Forbes Insights and KPMG "Global CEO Outlook," 84 percent of CEOs are concerned about the quality of the data they are basing their decisions on. As the world is becoming even more data-driven, it becomes critically important for business and data analytics to have the right data, in the right form, at the right time so that business analytics can turn it into accurate insights. A poor quality data destroys business value. According to Gartner's Data Quality market survey, the average financial impact of poor data on businesses is about $15 million per year. These expenditures are not only financial, businesses can see loss of reputation, missed opportunities and higher-risk decision making because of poor quality of data.
The Data Quality horrors likely to worsen as information environments become increasingly complex. Organizations of all sizes and complexity encounter data quality issues. Organizations having multiple functional units and operations spread across multiple geographies with voluminous customers, employees, suppliers and product/services are facing more severe data quality issues. The need for quality data is as important as ever today and this can be only achieved by embarking Data Quality improvement journey. Many organizations struggle to successfully propose the program for sustainable data quality improvement. A business case for data quality improvement must start and end with the business outcome. A successful Data Quality business case must address the key components necessary to achieve the business vision, such as financial performance, operational performance, legal and regulatory compliance and customer experience.
Once the scope of the business case is agreed, target state of Data Quality should be defined to achieve business improvements. A successful execution of Data Quality program demands executive sponsorship and formation of Data Governance Committee. The committee shall include nominations from executive leadership, all the business functions including IT and Operations. The Data Governance committee being ultimate decision taking authority decides the ownership, process and assigns Team to carry-forward the program. The team, which is composed of data owners, stewards & IT, further engage in recording data quality issues, identifying root cause, resolve the issues and build tools to measure & monitor the Data Quality. It is the collaboration between the teams that decide the success of Data Quality improvement program.
However, a Data Quality improvement program ensuring high-quality data should adhere to the 4-step approach:
Data Discovery: Organization has thousands of data elements & not all data elements directly influence business. It is important to perform a holistic assessment to identify business-critical data elements that effects business. Critical data elements are the ones, which appear as part of a business process, MIS/Analytic report, KPI, operational parameter or a control. Capability should be built to identify the critical data elements by recording systems, processes & teams associated with the data elements, which has direct impact on business performance. The critical data elements identified so shall become candidate for Data Quality improvement program.
Data Management: For managing the organization data, post-discovery, the data should be categorized to plan separate data management treatment. Identified data should be classified into master data, transaction data & reference data for its most effective and efficient use. Diverse data standards of the enterprise, standardized definitions of data attributes & data quality rules should be defined and recorded. Assets like Enterprise data dictionary, KPI workbook should be created &updated whenever there is an addition or change to the data definitions. Guidelines should be created for data creation, consumption, data archival & deletion as well.
Metadata Management: It involves instituting policies and processes to ensure information integrity, information access, sharing of information across the organization and analysis. Metadata management enables Data Quality improvement program to increase contextual understanding, transparency, availability, consistency, security and usability of data. Centralized library for Business metadata, Technical metadata and Operational metadata should be created & maintained by IT team along with data owner/steward.
Data Quality Management: Performing stewardship, change management and stakeholder management are the key activities of data quality management. To enable this organization should build capability to diagnose data quality issues, correct them & monitor the solution effectiveness for achieving sustainable Data Quality. This include building DQ Engine for assessment& reporting, automation of data cleansing jobs and deploying validations at the source of data entry.
Improving data quality can be a daunting task however, its benefits are manifold. It ensures good quality data, which empowers business insights, strengthens organization's competitive standing and encourage critical business objectives. The rewards of good quality data can be ripped in terms of improved customer experience, confidence in decision-making and lowering operational costs.
Abhishek Pal
Abhishek Pal is a techno-functional professional having over 17 years of rich experience in Information Technology (IT), Program Management, Analytics and Enterprise Data Management & Governance. Presently the Sr. Program Manager at Tata Communications Ltd., he has strong technical background in Systems/Application Architecture, EDM, Data Science and Big Data space.
The Data Quality horrors likely to worsen as information environments become increasingly complex. Organizations of all sizes and complexity encounter data quality issues. Organizations having multiple functional units and operations spread across multiple geographies with voluminous customers, employees, suppliers and product/services are facing more severe data quality issues. The need for quality data is as important as ever today and this can be only achieved by embarking Data Quality improvement journey. Many organizations struggle to successfully propose the program for sustainable data quality improvement. A business case for data quality improvement must start and end with the business outcome. A successful Data Quality business case must address the key components necessary to achieve the business vision, such as financial performance, operational performance, legal and regulatory compliance and customer experience.
Once the scope of the business case is agreed, target state of Data Quality should be defined to achieve business improvements. A successful execution of Data Quality program demands executive sponsorship and formation of Data Governance Committee. The committee shall include nominations from executive leadership, all the business functions including IT and Operations. The Data Governance committee being ultimate decision taking authority decides the ownership, process and assigns Team to carry-forward the program. The team, which is composed of data owners, stewards & IT, further engage in recording data quality issues, identifying root cause, resolve the issues and build tools to measure & monitor the Data Quality. It is the collaboration between the teams that decide the success of Data Quality improvement program.
However, a Data Quality improvement program ensuring high-quality data should adhere to the 4-step approach:
Data Discovery: Organization has thousands of data elements & not all data elements directly influence business. It is important to perform a holistic assessment to identify business-critical data elements that effects business. Critical data elements are the ones, which appear as part of a business process, MIS/Analytic report, KPI, operational parameter or a control. Capability should be built to identify the critical data elements by recording systems, processes & teams associated with the data elements, which has direct impact on business performance. The critical data elements identified so shall become candidate for Data Quality improvement program.
Data Management: For managing the organization data, post-discovery, the data should be categorized to plan separate data management treatment. Identified data should be classified into master data, transaction data & reference data for its most effective and efficient use. Diverse data standards of the enterprise, standardized definitions of data attributes & data quality rules should be defined and recorded. Assets like Enterprise data dictionary, KPI workbook should be created &updated whenever there is an addition or change to the data definitions. Guidelines should be created for data creation, consumption, data archival & deletion as well.
Metadata Management: It involves instituting policies and processes to ensure information integrity, information access, sharing of information across the organization and analysis. Metadata management enables Data Quality improvement program to increase contextual understanding, transparency, availability, consistency, security and usability of data. Centralized library for Business metadata, Technical metadata and Operational metadata should be created & maintained by IT team along with data owner/steward.
Data Quality Management: Performing stewardship, change management and stakeholder management are the key activities of data quality management. To enable this organization should build capability to diagnose data quality issues, correct them & monitor the solution effectiveness for achieving sustainable Data Quality. This include building DQ Engine for assessment& reporting, automation of data cleansing jobs and deploying validations at the source of data entry.
Improving data quality can be a daunting task however, its benefits are manifold. It ensures good quality data, which empowers business insights, strengthens organization's competitive standing and encourage critical business objectives. The rewards of good quality data can be ripped in terms of improved customer experience, confidence in decision-making and lowering operational costs.
Abhishek Pal
Abhishek Pal is a techno-functional professional having over 17 years of rich experience in Information Technology (IT), Program Management, Analytics and Enterprise Data Management & Governance. Presently the Sr. Program Manager at Tata Communications Ltd., he has strong technical background in Systems/Application Architecture, EDM, Data Science and Big Data space.