The potential of Data-Supported Decision Making in Educational Institutions
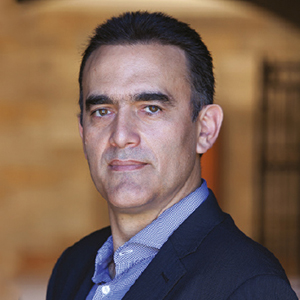
Abelardo Pardo, Associate Professor The University of Sydney
The ubiquitous presence of technology mediating an increasing number of interactions and processes has translated into a world in which data is everywhere. This data, and the possible ways in which it is being used are both increasing at unprecedented rates. Higher education institutions are not immune to this tread. In fact, their operational characteristics make them ideal contexts where data is collected and analysed to derive actionable knowledge affecting their core activities. Most of the data reporting has occurred so far at the level of upper management and, at least in the Australian context, due to strict government regulations. Institutions must collect comprehensive information about the progress of their students within the institution and report it at regular intervals to the designated bodies. Special units, either as part of the IT infrastructure, or as a separate entity typically labelled business intelligence has been performing these duties up to now. But these clearly delineated procedures are about to be seriously disrupted.
Data is also collected at the level of educational programs. Student scores are now stored for every assessment task, every written report, every quiz, and every midterm exam. Information about the trajectories that students follow through an increasingly varied set of programs is also being tracked. Up until now, this data was used solely for administrative purposes directly related with the academic context. But, the commoditization of data analytic techniques allows educational institutions to use this data to study how students evolve within the institutions, identify those students most likely to succeed or abandon the institution, or even acquiring a detailed characterisation of those students in high school that can be influenced when choosing their next education stage. The big paradigm shift is that all these analysis have a direct connection with revenue streams.
The increasing competition in the post-secondary sector is also leading to explore how to improve the student experience through personalisation. Ideally, students should be exposed to the resources, engage in activities, and participate in those interactions that are most adequate to maximise their academic achievement. This personalisation is a trend that is well under way in other contexts such as online retail, entertainment, marketing, etc. But in learning, this level of personalisation requires comprehensive data sets about student behaviour. Interestingly, institutions are already collecting massive amounts of data about wireless usage, physical presence in libraries and laboratories, and even engagement with a wide variety of online platforms (sessions in the learning management systems, discussion forums, engagement with recorded lectures and so on)
The combination of these three information levels (institutional, academic program, and every day interaction with electronic resources) should ideally translate into institutions that identify students needs well before they join the institution, adapt their portfolio of programs to suit their needs, and provide a fully personalised experience all throughout their academic experience. However, although technically possible, this vision is far from reality and one of the obstacles preventing its fulfilment is the fragmentation of data sources.
Educational institutions are complex organisational structures that require a combination of technical solutions at different level. As a consequence, the data is collected and stored across a wide variety of platforms and formats typically integrated into different organisational units. The contrast between these rigid and usually siloed institutional units and the need for a holistic approach to data management causes significant friction.
The design of a data-driven university requires a holistic approach. Institutional leaders must be aware of the emerging data ecosystem that is multi-modal and fragmented and articulate their vision to deploy horizontal structures that are sensitive to all stakeholders. Institutional reporting must be maintained and enhanced, academic units at different levels must have data about how students traverse the existing programs, and academics must have comprehensive information about how students are performing in their day-to-day activities. And, this data must be available through simple, fully integrated and secure platforms. We are heading into an era where students will end up demanding this type of service as a way to increase their chances of success and make the most of their education. Educational institutions cannot afford to ignore this need.
The vision is simple to describe, simple to understand, but significantly complex to implement. A cultural change is needed to fully embrace a mentality that acknowledges the potential of data-supported decision making in educational institutions to bring improvements that will ultimately translate into more fulfilling experiences to students.
Abelardo Pardo
Abelardo Pardo specialises in technology-enhanced learning with emphasis on learning and behavioural analytics, computer supported collaborative learning, and personalization of learning experiences. He is a member of the executive committee of the Society for Learning Analytics Research. In his (scarce) spare time, he maintains the blog T2T - Techies meet Teachers - which focuses on issues related to technology when used in learning experiences.
Data is also collected at the level of educational programs. Student scores are now stored for every assessment task, every written report, every quiz, and every midterm exam. Information about the trajectories that students follow through an increasingly varied set of programs is also being tracked. Up until now, this data was used solely for administrative purposes directly related with the academic context. But, the commoditization of data analytic techniques allows educational institutions to use this data to study how students evolve within the institutions, identify those students most likely to succeed or abandon the institution, or even acquiring a detailed characterisation of those students in high school that can be influenced when choosing their next education stage. The big paradigm shift is that all these analysis have a direct connection with revenue streams.
The increasing competition in the post-secondary sector is also leading to explore how to improve the student experience through personalisation. Ideally, students should be exposed to the resources, engage in activities, and participate in those interactions that are most adequate to maximise their academic achievement. This personalisation is a trend that is well under way in other contexts such as online retail, entertainment, marketing, etc. But in learning, this level of personalisation requires comprehensive data sets about student behaviour. Interestingly, institutions are already collecting massive amounts of data about wireless usage, physical presence in libraries and laboratories, and even engagement with a wide variety of online platforms (sessions in the learning management systems, discussion forums, engagement with recorded lectures and so on)
The combination of these three information levels (institutional, academic program, and every day interaction with electronic resources) should ideally translate into institutions that identify students needs well before they join the institution, adapt their portfolio of programs to suit their needs, and provide a fully personalised experience all throughout their academic experience. However, although technically possible, this vision is far from reality and one of the obstacles preventing its fulfilment is the fragmentation of data sources.
Educational institutions are complex organisational structures that require a combination of technical solutions at different level. As a consequence, the data is collected and stored across a wide variety of platforms and formats typically integrated into different organisational units. The contrast between these rigid and usually siloed institutional units and the need for a holistic approach to data management causes significant friction.
The design of a data-driven university requires a holistic approach. Institutional leaders must be aware of the emerging data ecosystem that is multi-modal and fragmented and articulate their vision to deploy horizontal structures that are sensitive to all stakeholders. Institutional reporting must be maintained and enhanced, academic units at different levels must have data about how students traverse the existing programs, and academics must have comprehensive information about how students are performing in their day-to-day activities. And, this data must be available through simple, fully integrated and secure platforms. We are heading into an era where students will end up demanding this type of service as a way to increase their chances of success and make the most of their education. Educational institutions cannot afford to ignore this need.
The vision is simple to describe, simple to understand, but significantly complex to implement. A cultural change is needed to fully embrace a mentality that acknowledges the potential of data-supported decision making in educational institutions to bring improvements that will ultimately translate into more fulfilling experiences to students.
Abelardo Pardo
Abelardo Pardo specialises in technology-enhanced learning with emphasis on learning and behavioural analytics, computer supported collaborative learning, and personalization of learning experiences. He is a member of the executive committee of the Society for Learning Analytics Research. In his (scarce) spare time, he maintains the blog T2T - Techies meet Teachers - which focuses on issues related to technology when used in learning experiences.